What is lung adenocarcinoma:
Lung adenocarcinoma is the most common malignant tumor arising in the lungs.
Such tumor is composed of out of control epithelial cells that proliferate in lung tissues in an unregulated way and accumulate mutations at the DNA.
This disease in most cases originates in alveolar and bronchial tissues, but if not detected and treated in time it can metastasize, so it affects other tissues such as lymph nodes.
The therapeutic course to treat such disease varies depending on several factors such as the stage of the tumor, the patient’s clinical history, and the time at which the diagnosis is made.
Today there are new possible approaches that also involve the use of artificial intelligence associated with animal models, such as murine one.
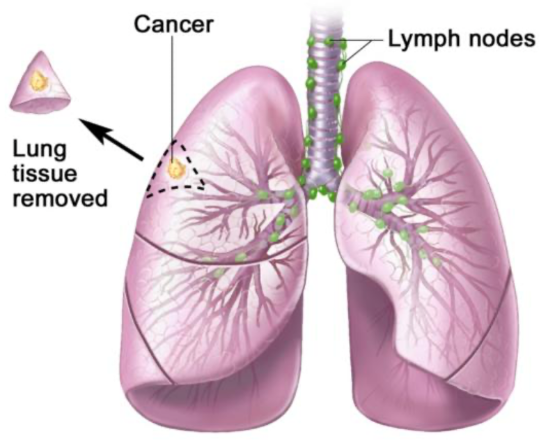
GEMMs and GLASS-AI:
Genetically engineered mouse models (GEMMs) used in the preclinical setting of lung adenocarcinoma turn out to be extremely useful in studying the molecular factors that determine tumor characteristics such as:
- its formation;
- tumor progression;
- the development of therapeutic resistance of the tumor.
Â
However, the analysis of GEMMs requires time and some academic background to ensure accuracy and consistency. Researchers to achieve a more accurate and standardized analysis use an image analysis tool known as Grading of Lung Adenocarcinoma with Simultaneous Segmentation by Artificial Intelligence (GLASS-AI); it is used to classify, segment and analyze tumors in preclinical models of lung adenocarcinoma. The integration of studies by researchers and the use of GLASS-AI emphasized the lack of regulation of some protein pathways in lung adenocarcinomas. The study conducted aims to demonstrate the benefits of using GLASS-AI in preclinical models of lung adenocarcinoma integrated with the integration of machine learning and molecular biology techniques to study the molecular pathways underlying cancer progression.
An important step occurred in 2017, when the U.S. Food and Drug Administration (FDA) approved the use of whole slide scanners in clinical pathology. This enabled rapid sharing of digital pathology images even in the pre-clinical setting.
Such images have increased the work efficiency of pathologists, but the fact that they are digitized allows collaboration among geographically distant groups.
In addition, advances in image processing have made possible new applications that can help in the histopathologic analysis of slides, particularly in the field of oncology.
These applications make it possible to quickly perform time-consuming tasks such as segmentation of nuclei and tumors, analysis of histologic staining or quantification of tumor-associated or tumor-infiltrating immune cells.
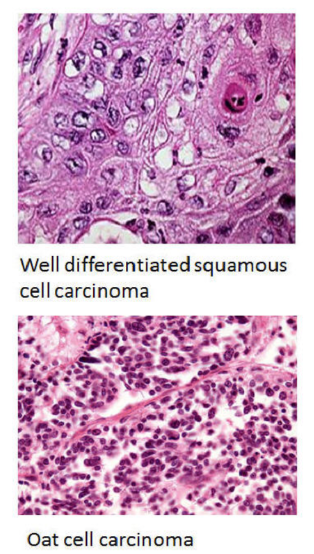
In many of these studies, the accuracy of the machine learning model was matched by analyses performed by experienced researchers. Applications of machine learning in preclinical research present an excellent opportunity to improve and accelerate analyses of the experimental data produced.
The application of machine learning models will likely revolutionize the way data are analyzed. This may help clinicians by providing rapid image screening and high-resolution analysis that can help detect features not reported by the human eye.
The study in question, published in “Nature“, was carried out based on a neural network specially programmed for the classification of lung adenocarcinomas in genetically engineered mouse models (GEMMs) that provides very accurate identification and analysis of tumor heterogeneity.
This new approach can be integrated with molecular biology techniques already widely used to develop an understanding of the molecular factors that determine the progression and heterogeneity of the tumor itself.
For example, localized loss of specific proteins regulation together with gene mutations drive toward tumor progression.
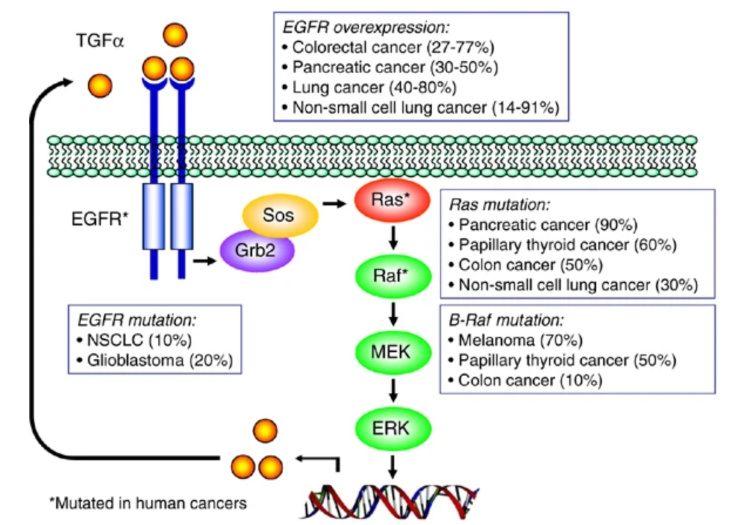
The use of these models increased the ability to accurately predict molecular tumor markers using histological images. In addition, it could be used to mark collected specimens for further molecular characterization and even provide greater precision in diagnostics and therapeutics.